Remote Sensing Technologies are being used to determine the physical properties of land and what it is used for. Deforestation/reforestation, illegal logging, safety of critical infrastructure like power lines and railway lines, and vegetation health are among the many use cases for this technology. Although there are excellent products built on top of free satellite imagery like Landsat 8, MODIS or Sentinel 2, these products may not be sufficient in terms of timeliness and resolution. Our suite of algorithms based on fusion of multiple modalities are focused on using these satellites in combination with higher resolution data. The high location revisit rate of SkySat and PlanetScope satellite constellations can add value to this analysis. Our solution is available on our scalable cloud platform where we offer our customers deforestation and vegetation expansion monitoring as an online analytical service.
Our solution exploits multimodal data fusion of satellite data of various resolutions and has been used successfully in multiple cases, proving its robustness and value to the customers. Some examples are detection of illegal logging, automated monitoring of vegetation growth in power line transmission corridors and in railway corridors, and spruce beetle infestation damage assessment. How does our solution work?
Materials and objects may exhibit similar spectral characteristics in the visible to near infrared range, but possess different backscattering properties on the wavelengths used by SAR satellites, such as provided by Sentinel 1. Combining spectral information from multispectral imagery together with polarimetric SAR information significantly improves the detection and segmentation of vegetation in the areas of interest. At SpaceKnow, we developed a novel approach for enhanced change detection by combining radar and optical satellite measurements and by performing data fusion using state-of-the-art processing and computer vision techniques. The key to success lies in exploiting both supervised models based on Deep Neural Networks (DNN) for precise pixel-wise analysis (semantic segmentation) as well as unsupervised change algorithms processing whole batch of time-stamped imagery to further enhance the resilience to false positives and false negatives (see Figure 1). The proposed data fusion integrates multiple sources of radar and optical data that are complementary to each other in terms of spatial, spectral, and temporal resolution. This combination of complementary information from radar and multispectral imagery is enabled for fully automated execution without any human-in-the-lop.
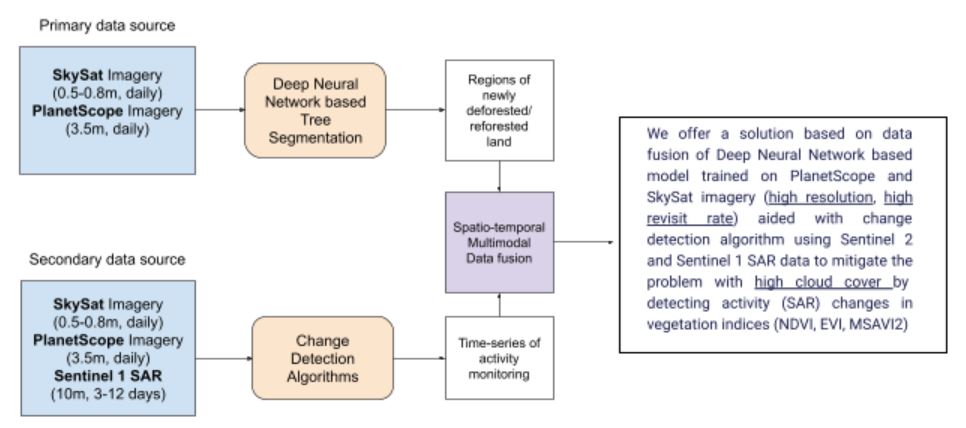
Our vegetation and tree detection models based on DNNs make full use of the 0.5m/pixel to 3.125m/pixel resolution multispectral imagery provided by Planet (see Figure 1 – primary data source). Although at first sight it may seem redundant to develop algorithmic solutions for both PlanetScope and SkySat imagery (both data sources offer high revisit rates), the ability to be flexible in scaling to different levels of detail allows us to use a single solution, single analytical service for locations of multiple sizes. For example, both country-sized polygons for deforestation detection as well as narrow corridors for nearby critical infrastructure where high resolution is important can be analyzed using this solution.
This computer vision analysis allows us to clearly distinguish between areas covered with dense vegetation (mainly trees and forests) and other areas. This analysis is available almost daily, due to high location revisit rates with bad weather and cloud coverage being the only limiting factor, common to optical imagery. It is therefore necessary to handle cloudy images appropriately. Discarding cloudy images based simply on per scene metadata is not an effective solution and in time-critical applications leads to missing valuable data points. Therefore, as an inherent part of our change detection algorithms we incorporated another DNN based model that provides pixel precise cloud masks. Such enhancement increases the robustness of the whole solution (see Figure 1 – secondary data source).
We carefully evaluated our solution in many different use cases. For precise farmland monitoring, as for example in Figure 2, we further complemented our vegetation analysis with building detection, aiming to distinguish residential housing and utility buildings like greenhouses.
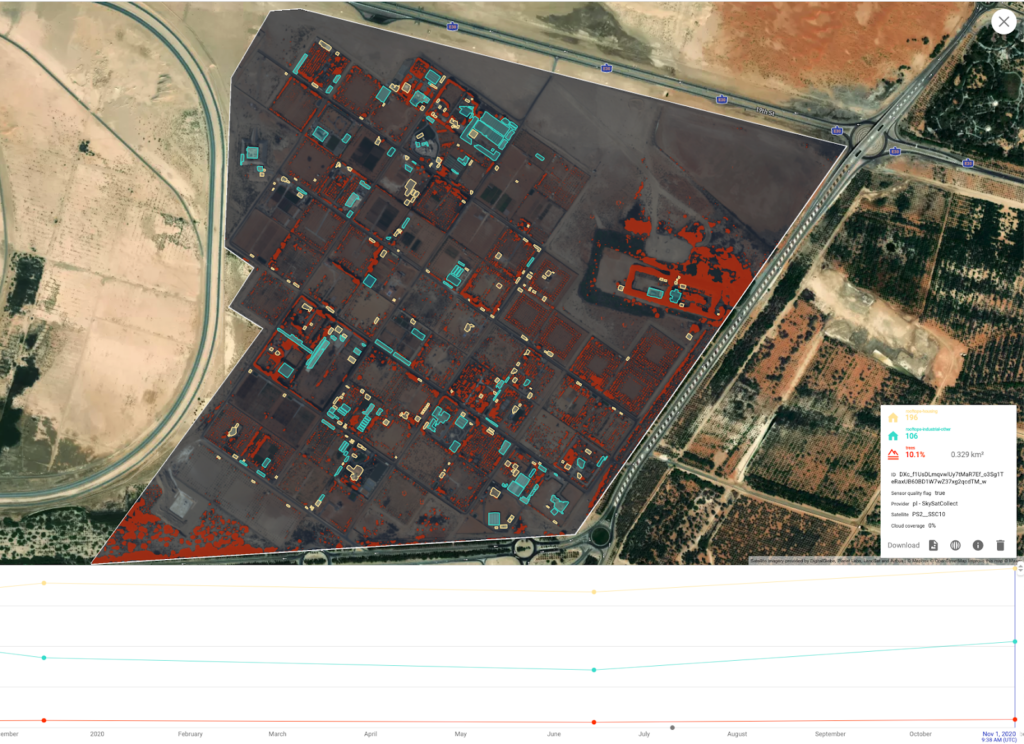
Vegetation expansion near power lines or within railway corridors represents a potential hazard for the whole distribution network or for the transportation system. Using our cloud based solution we can automatically monitor large areas and thousands of kilometres of power lines or corridors per day. To reliably identify places of potential risk, satellite monitoring has to precisely measure the trend in vegetation expansion over relatively short time intervals, i.e. the temporal aspect is important, including the confirmation of detections and changes in time. In Figure 3 we show how even the 10m resolution of Sentinel 1 SAR data can be used to aid the timeliness of our solution.
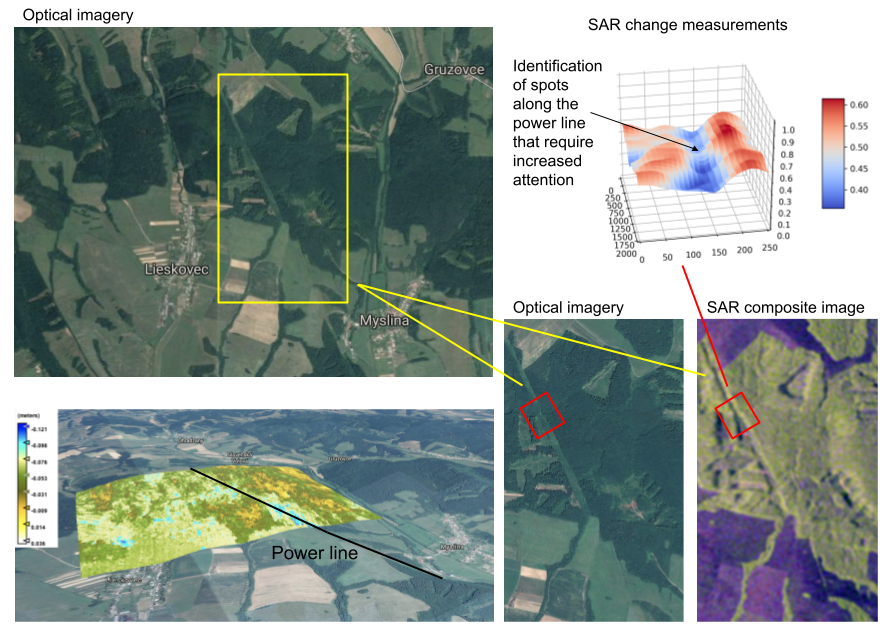
Another important use case concerns deforestation and illegal logging. In order to be effective when detecting and identifying illegal logging, resolution and revisit rates play a key role. Analyzing large, even country-sized regions with PlanetScope and then having the ability to tip & cue into areas of interest where alerts are triggered with higher resolution SkySat imagery ensures unprecedented precision and reliability. As this monitoring is happening in near real-time, with every new incoming image, changes are automatically evaluated and previous detections confirmed or discarded (see Figure 4 for illustration of the confirmation mechanism).
At SpaceKnow we focus on full automation & scalability. We build our solutions across multiple modalities and fuse every possible information source to provide our customers answers to their questions. Data quality is important and we utilize any additional metadata indicating image quality or missing and invalid pixels to improve our solution. As a result, we are able to enhance our detection confirmation mechanisms and clearly indicate the robustness of our solution to the customer.
This blog provides a deep look into our solution for deforestation and vegetation monitoring. Interested in learning more? Reach us at info@spaceknow.com or www.spaceknow.com.
Written by Michal Reinstein on behalf of SpaceKnow.